New publication from Dr. Saviz Moghtadernejad and Yuchuan Jin in the Journal of Infrastructure Systems!
Prof Bryan Adey's Chair of Infrastructure Management congratulates Dr. Saviz Moghtadernejad and Yuchuan Jin on the publication of the article "Estimating the Values of Missing Data Related to Infrastructure Condition States Using Their Spatial Correlation".
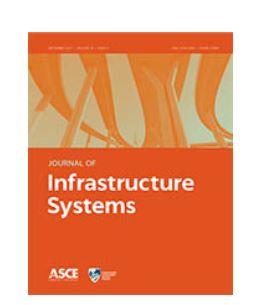
In this article, Dr. Saviz Moghtadernejad and Yuchuan Jin examine the efficiency of different models that use the spatial correlation of infrastructure assets in predicting the value of missing data. The models include univariate and multivariate Kriging, a hybrid artificial neural network (ANN)-Kriging model, and the bidirectional long short term memory (bi-LSTM) neural network, which can model the data with spatial correlation or a sequential relationship. The results confirm that the condition indicator values can be estimated with reasonably low levels of error.
Abstract:
Estimating the Values of Missing Data Related toInfrastructure Condition States UsingTheir Spatial CorrelationSaviz Moghtadernejad, Ph.D.1; Yuchuan Jin2; and Bryan Tyrone Adey, Ph.D.3Abstract:Infrastructure managers consistently monitor the condition of their assets to predict their deterioration speed and determine theoptimal time to execute preventive interventions. However, despite the recent progress in more frequent and accurate monitoring of assets andstorage of the related results, in practice, real-world data often contains errors and discrepancies such as missing data or faulty entries. Thisproblem can happen owing to collection errors during routine inspections or inconsistency of data storage formats in different years. Becausethe quality of data plays a significant role in the accuracy of deterioration prediction and the resulting intervention programs, it is important toimprove condition state predictions by imputing the values of missing information. This paper examines the efficiency of different models thatuse the spatial correlation of infrastructure assets in predicting the value of missing data. The models include univariate and multivariateKriging, a hybrid artificial neural network (ANN)-Kriging model, and the bidirectional long short term memory (bi-LSTM) neural network,which can model the data with spatial correlation or a sequential relationship. The results confirm that the condition indicator values can beestimated with reasonably low levels of error.
The Infrastructure Management Group mission is to improve the construction and management of infrastructure. For more information visit our webpage